Updated: September 24, 2024
Top Data Quality Management Tools
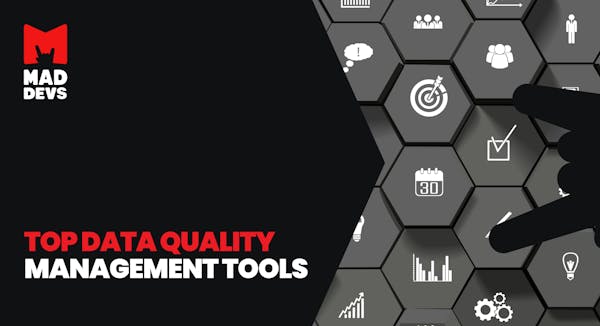
Nowadays, organizations heavily rely on data to make informed decisions, drive business strategies, and deliver exceptional customer experiences. However, the increasing volume, velocity, and variety of data pose significant challenges in ensuring its accuracy, reliability, and integrity. This is where data quality management comes into play.
Data quality management refers to structured processes, methodologies, and tools designed to assess, improve, and maintain data quality throughout its lifecycle. It encompasses various aspects such as data validation, profiling, cleansing, enrichment, and governance.
In this article, we explore the significance of data quality management, the methods and tools involved, and its implications for organizations seeking to unlock the full potential of their data assets. By prioritizing data quality, businesses can gain a competitive edge, make well-informed decisions, and improve business outcomes.
What is data quality?
Data quality refers to the process of ensuring that data suits an organization's specific needs. Frequent issues can manifest in various ways, such as data duplication, incomplete or inconsistent data, inaccuracies, lack of clear definition, disorganized data, and inadequate data security measures.
Assessments of data quality are conducted by analysts who interpret data quality metrics, calculate an overall score, and provide organizations with a percentage representing data accuracy. A low score indicates poor-quality data, which can negatively impact decision-making, potentially harming the organization.
Effective data governance follows criteria for ensuring data harmonization, establishes data usage policies, and addresses inconsistencies and inaccuracies that could undermine data analytics accuracy and regulatory compliance.
6 criteria for measuring the quality of data
These criteria are: accuracy, completeness, consistency, timeliness, validity, and uniqueness. They help ensure data is reliable and suitable for use in analytics, reporting, and decision-making. Here is a detailed explanation of each:
- Accuracy: Data should correctly represent real-world values or events without errors or distortions.
- Completeness: All necessary data should be available, with no missing elements that are required for analysis or decision-making.
- Consistency: Data should be uniform across systems and sources, without conflicting information or discrepancies.
- Timeliness: Data should be up-to-date and available when needed, ensuring relevance for the task or analysis at hand.
- Validity: Data must conform to defined formats, structures, and rules, ensuring it meets set standards and constraints.
- Uniqueness: Data should be free of duplicate entries, ensuring that each record is distinct and properly represented.
What are data quality tools?
Data quality tools are software applications or solutions designed to help organizations assess, improve, and maintain the quality of their data. These tools provide functionalities and features to address various aspects of data quality, such as accuracy, completeness, consistency, validity, and integrity.
Here are some standard capabilities offered by tools for quality data:
- Data profiling. Tools analyze data content, structure, and quality, generating statistical summaries, detecting patterns and anomalies, and providing insights on data types, distributions, and completeness.
- Data cleansing. Tools identify and resolve data errors, inconsistencies, and redundancies. They standardize formats, remove duplicates, correct misspellings, validate against rules, and transform data.
- Data validation. Tools apply predefined rules to check data for accuracy, integrity, and compliance, highlighting non-compliant data.
- Data matching and deduplication. Tools identify and consolidate duplicate records, ensuring a single, accurate data view.
- Data standardization. Tools standardize data formats and values, ensuring consistency. They apply predefined rules, transforming data into a standard format and eliminating variations in naming conventions and abbreviations.
- Data enrichment. Tools integrate external data sources (e.g., demographics, geolocation) to enhance datasets, providing additional insights and context.
- Data monitoring and alerts. Tools enable automated data quality monitoring, setting thresholds, and generating alerts for issue detection and proactive resolution.
- Data reporting and dashboards. Tools generate quality reports, metrics, and visualizations. They offer interactive dashboards and customizable reports for a comprehensive view of data quality, progress tracking, and improvement insights.
- Data governance support. Tools aid data governance by establishing policies, defining standards, managing metadata, and facilitating stakeholder collaboration for data integrity, compliance, and accountability.
Tools offer some standard capabilities, but the specific functionalities may vary depending on the vendor. Organizations can choose the tools that best align with their data quality requirements and business objectives. However, remember the limitations of these tools, which should be considered when choosing them. Please note some restrictions that data management tools can't do:
📖 Test management tools aid in locating and controlling errors, enabling efficient software development. Learn more reviews of five top tools, comparing features to help you choose the best for your test cycle.
- Data creation. Management tools rely on existing data sources and cannot create data where it doesn't exist. They work with the data available to them for analysis and improvement.
- Contextual understanding. While solutions provide valuable insights into data quality, they may lack the contextual understanding specific to industries, domains, or business requirements. Human expertise is necessary to interpret data within these specific contexts.
- Data strategy. These tools are within a broader data strategy framework. While they assist in implementing data quality practices, they must include a comprehensive data strategy encompassing data governance, management, and architecture.
- Data privacy and security. Although tools can identify specific data quality issues, they may not be able to address all privacy and security concerns relating to sensitive data. Organizations must implement additional measures to ensure data privacy and security.
- Decision-making. While data quality tools can provide valuable insights into data quality, they cannot make decisions or interpret data for decision-makers. To make informed decisions based on data insights, human judgment is required.
- Data ownership and responsibility. There is no assumption of responsibility for data quality issues by solutions. While they can aid in improving data quality, organizations remain responsible for ensuring data quality throughout the data lifecycle.
Understanding the capabilities and limitations of tools is crucial for organizations seeking to optimize their data quality initiatives. By leveraging software effectively and supplementing their functionalities with human expertise and a comprehensive data management strategy, organizations can unleash the full potential of their data assets, make informed decisions, and improve business outcomes.
How to choose the right data quality tool?
Choosing the right data quality tool is crucial for organizations seeking to improve their data quality. The key considerations for selecting the appropriate tool include identifying requirements, evaluating features and functionality, considering ease of use, integration capabilities, scalability and performance, vendor reputation and support, and assessing cost and return on investment (ROI). By carefully evaluating these factors, organizations can make an informed decision and implement a solution that aligns with their needs and drives improved data quality. Here are some important tips to help you with the selection:
- Assess and identify the specific data-related challenges your business is currently encountering.
- Gain a comprehensive understanding of the different tools available to help you overcome those challenges.
- Evaluate the advantages and disadvantages of each platform, considering how well they align with your specific needs and requirements.
- Consider each tool's pricing plans and costs, ensuring they fit within your allocated budget.
By following these steps, you can make an informed decision and select the most suitable software to effectively address your business's challenges.
In selecting data quality tools, what stakeholders need to be involved?
When selecting data quality tools, it is essential to involve key stakeholders from different departments and roles within an organization. The following stakeholders should be considered:
Chief Marketing Officer / Head of Marketing
High-level decision-makers should be involved to provide strategic direction and align the selection of data quality tools with the organization's overall goals and objectives. Someone who leads a marketing department, such as a CMO, VP of marketing, marketing manager, etc. CMOs rely on data for:
- Efficient tech stack
- ROI proof
- Customer-centricity push
- Insights & strategy iteration
- Creating more MQLs
- Personalized emails
- Email list management
- Lead scoring
- Marketing data analysis
- Fragmented customer personas
- Cross-channel & device user persona tracking
Head of Sales
Today, most sales departments utilize a broad tech stack and automated processes, gathering data from various sources like CRM and ERP systems. Data accuracy is vital for trustworthy figures, as sales reps' valuable time should focus on revenue-generating activities rather than data updates. However, maintaining clean data can be challenging despite preventive measures. Sales automation tools are practical when built on accurate data. A Head of Sales needs internal data for performance tracking, sales cycle analysis, and lead-to-customer conversion rates. Aligning sales and marketing data can be beneficial, but their data needs often differ. Some may need more data expertise, making complex data quality tools challenging to implement.
Data management team
This team is responsible for data governance, integration, and quality management. They understand the specific data requirements and challenges within the organization.
Head of IT Department
The IT department is occasionally responsible for the CRM, but only sometimes. Their main role is implementing and facilitating information flow, although they may not necessarily handle data accuracy processes. Understanding their existing practices is essential to understanding their needs for a data quality tool. There may be an unused tool already in place. Gaining buy-in from all team members is crucial. The Head of IT prioritizes a manageable tech stack, so the chosen tool should be easy to integrate or, ideally, require no changes to the existing set of technologies.
Other stakeholders. Your company may have other people affected by bad data, such as:
Business users. Representatives from various business units, such as marketing, sales, finance, and operations, should be involved to ensure that the selected tools align with their specific data needs and objectives.
Compliance and legal teams. In organizations with strict regulatory requirements, involving compliance and legal teams ensures that the selected tools meet legal and compliance standards.
By involving different stakeholders, organizations can gain valuable input, ensure comprehensive evaluation, and select data quality tools that best meet their specific needs and requirements.
Best data quality tools for your use
This part lists the top data quality tools that should be considered for effective data quality management.
Ataccama
Ataccama ONE is a comprehensive open-source data management platform that combines data governance, quality, and management functionalities into a unified AI-powered solution. With the support of hybrid and cloud environments, this tool empowers data teams to expedite their decision-making processes without compromising data trust, security, and governance. Integrating Ataccama ONE can help you streamline data operations, enhance data quality, and promote adherence to data governance standards, resulting in improved efficiency and secure business outcomes driven by data.
Features
- Allows business users to perform basic data quality tasks like running data profiling reports and viewing data quality metrics.
- Uses AI to automate data quality tasks and identify complex data quality issues.
- Аvailable as a cloud-based service, making it easy to deploy and scale.
- Exposes an open API, which allows you to integrate it with other applications and systems.
Pros
- Outstanding data profiling capabilities.
- User-friendly and adaptable.
- Offer rapid data processing capabilities.
Cons
- The configuration process can be time-consuming.
- Identifying error causes can be challenging.
- Technical support may be limited due to its open-source nature.
Overview
Ataccama has received 20% of its overall positive feedback from customers in the U.S. banking industry. The tool has helped banks to improve their data quality, governance, and lineage, which has led to several benefits, including:
- Increased data reliability and accuracy
- Improved decision-making
- Reduced compliance risk
- Increased efficiency and productivity.
Ataccama One has earned an overall rating of 4.6 out of 5 and is considered a high-value solution by many users. Here is an overview of customer feedback from different industries about Ataccama:
- Customers of banking and financial services highly praise Ataccama for its effective data quality and management capabilities. Ataccama One has enabled customers to manage better, govern, and improve their data quality. The platform is flexible, scalable, and easy to use, with good support for mobile device management (MDM) and reference data management (RDM).
- Healthcare industry customers have appreciated Ataccama's capabilities in handling complex healthcare data and improving data quality. Ataccama One has enabled customers to manage their data more efficiently and has improved visibility into data quality issues. The platform's unified approach to data management has proved particularly useful to healthcare providers.
- Retail industry customers of Ataccama have highlighted the platform's ability to manage large volumes of data, automate data quality processes, and enhance data governance. Customers have praised Ataccama's easy-to-use interface and powerful features, which have helped retailers make better-informed business decisions.
- Manufacturing industry customers of Ataccama have emphasized the platform's ability to handle complex supply chains and large volumes of data. Ataccama One has helped customers improve their data quality, provide better visibility into data quality issues, and streamline data management processes.
Precisely
Precisely is a powerful data quality tool that ensures data integrity through accurate and consistent data management. Also, this tool provides a range of data quality solutions, including Trillium for big data, Trillium data quality, and Trillium cloud. These solutions enable users to make informed and timely business decisions by gaining deep insights into their data. With Precisely, users can confidently rely on high-quality data for improved operational efficiency and decision-making.
Features
- Exposes an open API, which allows you to integrate it with other applications and systems.
- The impact analysis tool helps you understand the impact of data quality issues on your business processes so that you can prioritize your remediation efforts.
- Provides a comprehensive set of data quality metrics, so you can track the progress of your data quality initiatives and identify areas for improvement.
- The data quality dashboard offers a centralized view of your data quality metrics, so you can easily monitor the health of your data.
Pros
- Improved data quality.
- Increased compliance such as GDPR and CCPA.
- Reduced risk of data breaches and other data-related incidents.
- Improved efficiency.
Cons
- Difficult to use.
- Complex installation process and user interface (UI).
According to the reviews on Gartner by customers from different industries, Precisely is a highly appreciated data management and data quality tool. It earned an overall rating of 4.5 out of 5 and is considered a high-value solution by many users. Here is an overview of customer feedback from different industries about Precisely:
- Banking industry customers of Precisely have praised the tool's high reliability and accuracy, essential for managing large volumes of financial transactions. Customers also appreciate the scalability and flexibility of Precisely.
- Healthcare industry customers have praised the ease of use of Precisely, its ability to manage large volumes of data from different sources, and its data quality, which has improved the efficiency of its processes and enabled better patient care.
- Retail industry customers have highlighted Precisely's ability to simplify their data processes, identify inconsistencies, and improve overall data quality. Additionally, Precisely's user-friendly interface and customization features make it easy for retail teams to engage with and extract meaningful business insights.
- Manufacturing industry customers have praised Precisely's highly accurate data matching and merging capabilities, which have helped them keep their data sets organized for better supply chain management. Precisely's automation features have also helped streamline processes and simplify expense management.
SAP
SAP stands for Systems Applications and Products in Data Processing. It is a suite of integrated applications that helps businesses manage their core processes, such as finance, human resources, and supply chain management. SAP is one of the world's most popular enterprise resource planning (ERP) systems, with over 440,000 customers in more than 180 countries.
Features
- Includes several core modules like Financial Accounting (FI), Controlling (CO), Human Resources (HR), and Sales and Distribution (SD) that cover the essential business processes of an organization.
- SAP offers modules like CRM, EAM, and PLM to support specific business functions.
- Security: SAP is a secure platform that protects sensitive data. It includes some security features, such as user authentication, data encryption, and access control.
- Scalability: SAP can be scaled to meet the needs of businesses of all sizes. It can be deployed on-premises or in the cloud and scaled up or down as needed.
- Customization: SAP can be customized to meet the specific needs of each business. This customization can be achieved through various methods, such as ABAP programming, user exits, and function modules.
- Support: SAP has a large and experienced support team that can help businesses with implementation, training, and troubleshooting.
Pros
- Scalability to meet the needs of businesses of all sizes.
- Customization to meet the specific needs of each business.
- Integration with various other applications makes sharing data across the enterprise easy.
- Platform security that protects sensitive data.
- The support team helps businesses with implementation, training, and troubleshooting.
Cons
- SAP is a costly platform, both to purchase and to implement.
- Complex platform, challenging to learn and use.
- Constantly evolving platforms and businesses need to be prepared to upgrade systems regularly.
SAP Information Steward has earned an overall rating of 4.1 out of 5, indicating that its users consider the product highly valuable and effective. Here is an overview of customer feedback from different industries about SAP:
- Banking and financial services industry customers of SAP have praised the tool's ability to manage large volumes of complex financial data, maintain data quality, and enforce compliance. Customers also appreciate the scalable and customizable nature of SAP.
- Healthcare industry customers have praised SAP's ability to handle complex healthcare data, streamline data management processes, and improve the efficiency of their operations. SAP's advanced analytics and data visualization capabilities have helped healthcare providers make better-informed decisions and enhance patient care.
- Retail industry customers have highlighted SAP's capabilities in inventory management, supply chain optimization, and enhancing customer experiences. SAP's predictive analytics features have helped retailers identify trends and make informed business decisions.
- Manufacturing industry customers have outlined SAP's capabilities in managing complex supply chains, forecasting demand, and predicting production outcomes. SAP's analytics and data visualization features have helped manufacturers identify opportunities and pain points throughout their supply chains.
📖 Optimize your software development with powerful reporting tools. Gain insights, improve decision-making, and boost efficiency.
rg1
rg1 is a cloud-based data quality management solution developed by Redpoint Global. It helps organizations to improve the quality of their data by providing a range of features, including:
Features
- rg1 can automatically profile your data to identify potential issues, such as missing values, duplicate records, and incorrect data types.
- It can help you to clean your data by removing errors, correcting inconsistencies, and standardizing data formats.
- The tool can help you to validate your data by checking it against business rules and data dictionaries.
- It can help you track the lineage of your data, so you can understand where it came from and how it has been used.
- rg1 can help you implement data governance policies and procedures to ensure the quality of your data over time.
Pros:
- Cloud-based solution, so it is a convenient option for organizations with remote employees or needing to access their data from different locations.
- Scalable solution, so can be used to manage large datasets.
- Offers a wide range of features, including data profiling, data cleansing, data validation, data lineage, and data governance, so it is a powerful tool for organizations that need to improve the quality of their data.
- Easy to use, even for users with limited data quality experience.
Cons:
- Expensive solution.
- Has fewer integrations with other applications than other data quality management solutions.
- rg1's support is less widely available than other data quality management solutions.
Overview
According to customer reviews from Gartner Peer Insights, rg1 is:
- A data quality solution that helps organizations cleanse, transform, and integrate their data.
- A comprehensive solution that includes features for data profiling, parsing, standardization, cleansing, matching, monitoring, rule creation, and analytics.
- Easy to use and can be integrated with existing software systems.
Here are some reviews from Garter about rg1 by industry:
- IT Services: 32% of customers use rg1 to cleanse and integrate data from a variety of sources, including customer relationship management (CRM) systems, marketing automation platforms, and customer support systems.
- Healthcare and Biotech: 12% of customers use rg1 to cleanse and integrate data from a variety of sources, including electronic health records (EHRs), patient portals, and research databases.
- Media: 12% of customers use rg1 to cleanse and integrate data from a variety of sources, including advertising platforms, social media platforms, and CRM systems.
- Services (non-government): 12% of customers use rg1 to cleanse and integrate data from a variety of sources, including CRM systems, enterprise resource planning (ERP) systems, and human resources systems.
- Other: 32% of customers use rg1 to cleanse and integrate data from various sources, including retail systems, manufacturing systems, and financial systems.
Talend Data
Talend Data is a comprehensive data integration platform that helps organizations connect, manage, and transform their data. It provides a unified view of all data, making extracting insights and driving business decisions easy, regardless of where it resides.
Features
- Can connect to virtually any data source, including on-premises, cloud, and big data platforms.
- Includes several features for ensuring the quality of your data, such as data profiling, data cleansing, and data validation.
- Help you govern your data by providing a single view of all your data assets. It also includes features for managing data access, data security, and data lineage.
- It can transform your data in various ways, including cleansing, mapping, and enrichment.
Pros
- Offers different data integration, quality, governance, and transformation features.
- The drag-and-drop interface makes connecting to data sources, builds pipelines, and transforms data quickly.
- Scalable platform that can handle large volumes of data.
- Talend Data is a cost-effective solution for data integration. It is a subscription-based platform, meaning you only pay for the needed features.
Cons
- Complex to learn and use, especially true for users with limited data engineering experience.
- Documentation can be limited, making troubleshooting problems or answering questions difficult.
- The tool can be slow for large data sets, which can be a problem for organizations that must process large amounts of data in real-time.
Overview
Talend Data is a popular data integration platform that organizations of all sizes use. It is known for its wide range of features, including data integration, quality, governance, and transformation. Customers who have reviewed Talend Data on Gartner Peer Insights give it an average rating of 4.4 out of 5 stars. They appreciate the platform's ease of use, scalability, and cost-effectiveness.
Here are some additional details about Talend Data that are mentioned in the customer reviews:
- Talend Data is a good choice for organizations that need to integrate and transform large datasets. It is also a good choice for organizations that need to ensure the quality of their data.
- Talend Data could be better suited for organizations that must process large amounts of data in real-time. Learning and using it can also be complex, so it is not a good choice for organizations with limited data engineering experience.
Ataccama, Precisely, SAP, rg1, and Talend Data are crucial for ensuring accurate and reliable data, streamlining data operations, enhancing decision-making, improving customer satisfaction, enabling regulatory compliance, facilitating data integration, and supporting data governance.
These tools offer various benefits, including increased data reliability, improved decision-making, reduced compliance risk, increased efficiency, enhanced operational efficiency, and improved data governance. When selecting a data quality tool, organizations should carefully evaluate their requirements, consider features and functionalities, and weigh the pros and cons.
Prospects for the development and future of data quality management
The prospects for the development and future of data quality management are up-and-coming. Accurate and reliable data will become increasingly important as businesses become more data-driven. This is leading to a growing demand for data quality management solutions.
Here are some of the key trends that are driving the development of data quality management:
- The increasing volume, velocity, and variety of data are making it more challenging to manage data quality. Data quality management solutions are needed to help businesses ensure that their data is accurate and reliable.
- The rise of artificial intelligence (AI) is being used to automate many tasks in data quality management, such as data profiling, data cleansing, and data validation. This is freeing up data professionals to focus on more strategic tasks.
- Data governance is the set of policies and procedures ensuring data is managed consistently and competently. Data quality management is an essential part of data governance, and the two are often complementary.
The future of data quality management is bright. Accurate and reliable data will become increasingly important as businesses become more data-driven. This will lead to the further development of data quality management solutions and the adoption of these solutions by businesses of all sizes.
Conclusion
In conclusion, data quality tools are software solutions that assess, improve, and maintain data quality. They address accuracy, completeness, consistency, validity, and integrity. Tools offer features like profiling, cleansing, validation, enrichment, monitoring, integration, and governance.
To choose the right tool, consider specific challenges, and evaluate features, ease of use, integration, scalability, vendor support, and cost. If you need help implementing these tools, please contact us. Our specialists will contact you at a convenient time for you.
Data quality tools are essential for maintaining accurate data, automating management tasks, enhancing decision-making, improving customer satisfaction, ensuring compliance, enabling integration and analysis, and facilitating governance.
Tools can analyze and profile data, cleanse and validate it, monitor quality, integrate data, and support governance. However, they cannot create data, provide contextual understanding, replace a comprehensive data strategy, address all privacy and security concerns, make decisions, assume ownership, or interpret data for decision-makers.
Data quality management plays a vital role in ensuring accurate data. Prioritizing data quality gives organizations a competitive edge and drives improved business outcomes.
FAQ
How do data quality management tools help improve data accuracy and reliability?
Data quality tools enhance data accuracy and reliability through profiling, cleansing, validation, and standardization processes, ensuring consistent and error-free data for improved decision-making.
What features should I look for when selecting a data quality management tool?
When selecting a data quality management tool, consider features like data profiling, cleansing, validation, and enrichment. Look for capabilities in duplicate detection, standardization, and integration with various data sources. Ease-of-use, scalability, reporting, and data governance functionalities are also important. Additionally, consider compatibility with your existing systems, customization options, and vendor support to ensure the tool aligns with your specific data quality needs.
What level of technical expertise is required to use data quality management tools?
Data quality management tools vary in their complexity, but generally, a moderate level of technical expertise is required. Familiarity with data structures, profiling, validation rules, and data transformation concepts is beneficial. User-friendly interfaces and documentation can aid users with varying technical backgrounds.
How do data quality management tools measure and report on data quality metrics?
Data quality management tools measure and report on data quality metrics through predefined rules and criteria. They analyze data against these metrics, identifying issues and generating reports with insights on data completeness, accuracy, consistency, and other quality dimensions.