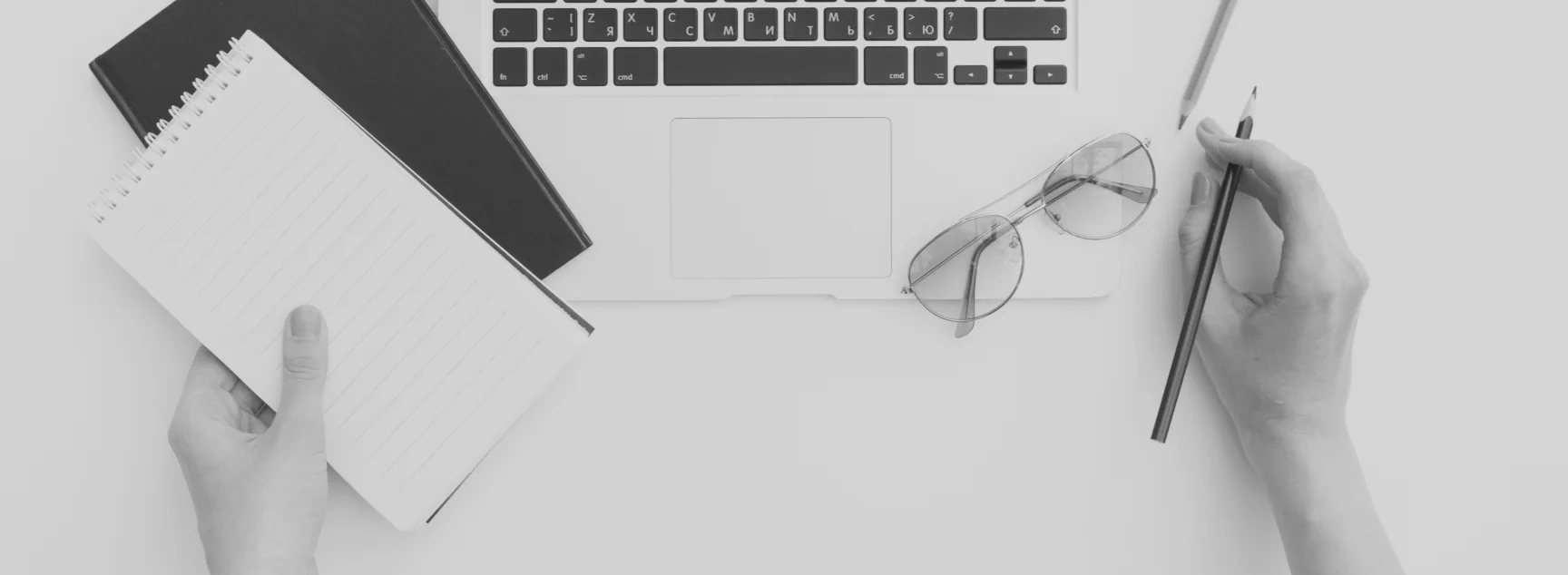
No Bad Questions About ML
Definition of Foundation models for AI
What are the foundation models for AI
This page will provide insights into foundation models, alternatively called general-purpose artificial intelligence or GPAI.
What are foundation models in AI
In AI, foundation models are large pre-trained neural networks models that form the basis for downstream tasks. Some of the examples include GPT, BERT, and T5. They learn general patterns and representations from extensive datasets to form a foundational understanding of language and data structures.
The key benefit of foundational models lies in their avoidance of starting from scratch, saving time and resources. Instead, they offer adaptability, allowing for further training or fine-tuning to address a wide range of specific problems.
How do organizations use foundation models?
After training, a foundation model leverages its knowledge to offer valuable insights to organizations and solve a wide range of problems. Some of its tasks include:
Natural language processing (NLP)
The foundation model recognizes context, grammar, and linguistic structures in NLP. Calibration for sentiment analysis helps with the assessment of customer feedback, online reviews, and social media posts.
This model can identify shapes, features, and patterns. Further fine-tuning enables automated content moderation, facial recognition, and image classification, generating new images based on learned patterns.
Audio or speech processingĀ
Recognizing phonetic elements, the model interprets voices and enhances communication through virtual assistants, multilingual support, voice commands, and transcription for accessibility and productivity.
Opportunities and risks of foundation models
Foundation models possess language, audio, and vision capabilities. Given their broad adaptability, foundation models hold the potential for diverse applications across various industries, including the following:
This paragraph was written based on the "On the Opportunities and Risks of Foundation Models" article. If you want to go deeper, you can read the full article here.
Healthcare
Foundation models offer cost-effective knowledge expansion in healthcare and biomedical research by leveraging diverse datasets for enhanced AI system interaction, potentially revolutionizing areas like drug discovery. However, there are risks, such as reinforcing biases in medical data and necessitating responsible usage considerations regarding data sources, privacy, interpretability, and regulation.
Law
In the legal field, foundation models aid in navigating complex narratives and standards supported by ample legal data. However, further advancements are needed for accurate, long-form document generation, and efficient adaptation is essential due to expert time costs. Privacy considerations and transparency in behavior are crucial, urging fundamental advancements for reliable generation accuracy.
Education
Foundation models hold promise in AI for education by enhancing understanding of student cognition and learning goals. Despite limited education data, combining external data and various approaches can expand application cases. Efficient resource use can lead to adaptive learning, but addressing emerging concerns and focusing on technology is crucial.
Among these concerns stated above, foundation models present numerous challenges:
Bias
Foundation models can inadvertently perpetuate and amplify biases in the training data. This can lead to unfair or discriminatory outcomes, especially if the training data reflects existing societal biases.
System constraints
Computer system limitations hinder scaling the model size and data quantity. Training foundation models demand significant memory resources, making it costly and computationally intensive.
Data dependency
Training foundation models often involves vast amounts of data, and this raises some privacy concerns. If the data used for training contains sensitive or personally identifiable information, there is a risk of privacy breaches.
Security concerns
Foundation models, like any software, may have security vulnerabilities that malicious actors could exploit. Ensuring the security of these models is crucial to prevent manipulation or unauthorized access.
Key Takeaways
- Foundation models are pivotal in diverse application scenarios such as natural language processing, computer vision, and audio processing.
- Organizations use trained foundation models for various tasks, excelling in NLP, computer vision, and audio processing. They offer insights for sentiment analysis, content moderation, and transcription.
- Foundation models, possessing multimodal capabilities, hold the potential for widespread applications in healthcare, law, education, and more. However, they come with challenges that organizations must navigate to harness their potential responsibly.