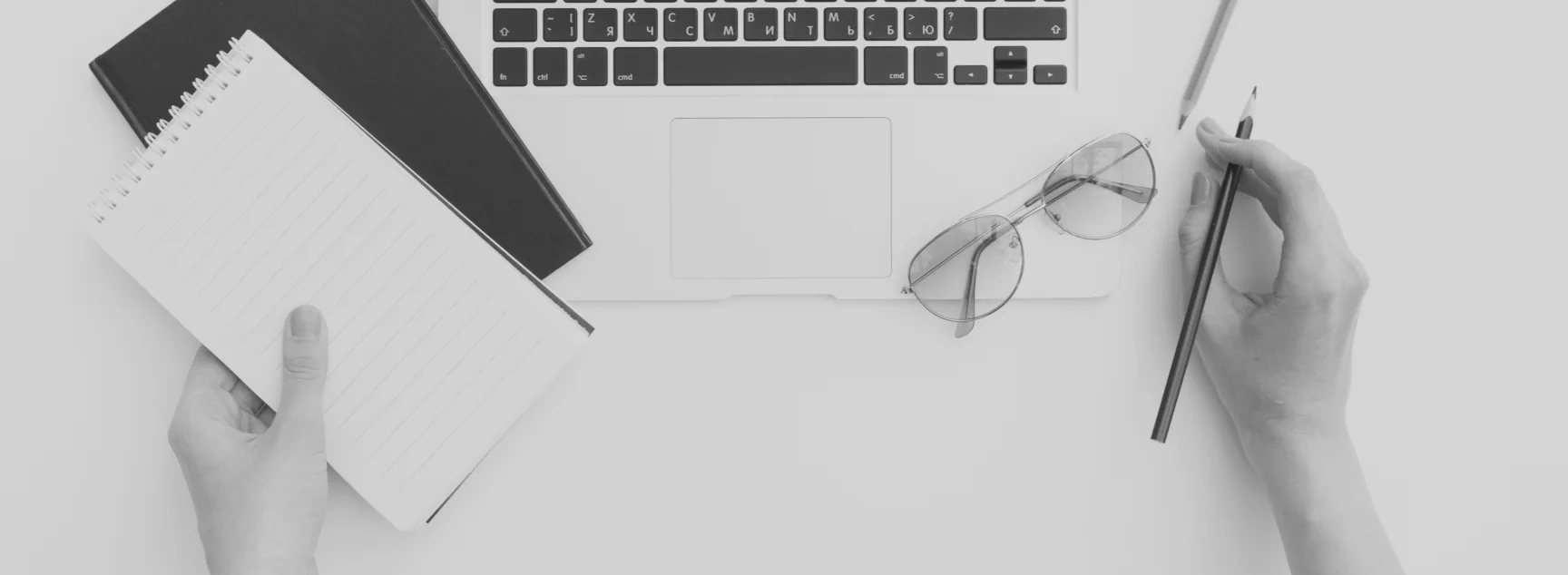
No Bad Questions About Data Management
Definition of Big data analytics
What is big data analytics?
Big data analytics is the process of examining datasets that are too large and complex to be analyzed through traditional means to uncover patterns, trends, and insights that drive decision making. Advanced tools and techniques are employed to accomplish this goal, including artificial intelligence, machine learning, and statistical models.
Businesses and organizations leverage big data analytics to optimize operations, improve customer experiences, and gain a competitive edge. The data analyzed comes from various sources, such as social media, IoT devices, transaction records, and sensors. By making sense of this vast information, companies can make more informed decisions and predict future trends with greater accuracy. Ultimately, big data analytics transforms raw data into actionable intelligence that enhances efficiency and innovation.
How does big data analytics work?
There are three broad steps in big data analytics: collection, processing, and analysis. These steps deal with large volumes of structured and unstructured data to extract meaningful insights.
- Data collection: From diverse sources such as websites, mobile applications, and connected devices.
- Processing: Cleaning, transforming, and organizing the data for analysis.
- Analysis: Various techniques, including machine learning algorithms, data mining, and predictive modeling, are applied to detect trends and correlations.
Big data security should be a high priority throughout each step to ensure no one receives unauthorized access to commit malicious acts. The results of big data analytics can be visualized with tools to help present the findings in an understandable format and enable decision makers to act on them. Another key element of big data analytics is constant review of the data and new analysis to refine predictions and improve business strategies over time.
What are the 5 V's of big data?
The five V's of big data, volume, velocity, variety, veracity, and value, define its key characteristics. They are a form of guidelines to help ensure that big data analytics delivers the expected benefits to decision makers.
- Volume: The vast amount of data generated daily that requires powerful storage and processing solutions.
- Velocity: The speed at which data is created and processed, often in real time.
- Variety: The different types of data, including structured (databases), semi-structured (JSON, XML), and unstructured (videos, social media posts).
- Veracity: The accuracy and reliability of data, which is crucial for making informed decisions.
- Value: The importance of extracting meaningful insights that drive business success and innovation.
The 5 V's provide a starting point for the collection and processing of data involved in big data analytics; however, there are 4 methods that guide the analysis step.
What are the 4 types of big data analytics?
Big data analytics consists of four main types: descriptive, diagnostic, predictive, and prescriptive analytics. These are methods that define how a business or organization understands and applies the data they collect.
- Descriptive analytics: Focuses on summarizing past data to provide insights into what has happened.
- Diagnostic analytics: Studies past data to determine why certain events occurred.
- Predictive analytics: Uses statistical models and machine learning to forecast future trends and potential outcomes.
- Prescriptive analytics: Suggests actions based on predictive insights to optimize strategies and decision making.
Each method delivers specific benefits, and businesses can combine them to gain a comprehensive understanding of their data for proactive planning.
Why is big data analytics important?
Big data analytics is important because it helps organizations make data-driven decisions that enhance efficiency and competitiveness. Perhaps the greatest benefit is that big data analytics helps businesses increase their revenue. By analyzing vast amounts of information, businesses can identify customer preferences, market trends, and operational inefficiencies.
Furthermore, big data and analytics are transforming supply chain management across several industries such as healthcare, finance, retail, construction, and manufacturing. The insights gained from analysis help improve processes and predict risks. Additionally, big data analytics enhances security by detecting fraud and cyber threats in real time. Governments and researchers also use it to address global challenges, such as climate change, disease outbreaks, and urban planning. Big data analytics empowers organizations to innovate, reduce costs, and create better products and services.
Key Takeaways
- Big data analytics examines large datasets that traditional analysis methods can't handle to uncover patterns, trends, and insights through AI, machine learning, and statistical models.
- The process involves data collection, preprocessing, analysis, and visualization.
- Big data is typically collected from various sources, including social media, IoT devices, and transaction records.
- The 5 V's of big data are volume, velocity, variety, veracity, and value, which provide a structure for collecting and processing the data.
- There are 4 methods employed in big data analytics: descriptive analytics, diagnostic analytics, predictive analytics, and prescriptive analytics, which all deliver specific benefits to businesses.
- Big data analytics helps businesses optimize operations, improve customer experiences, and stay competitive by creating better products.