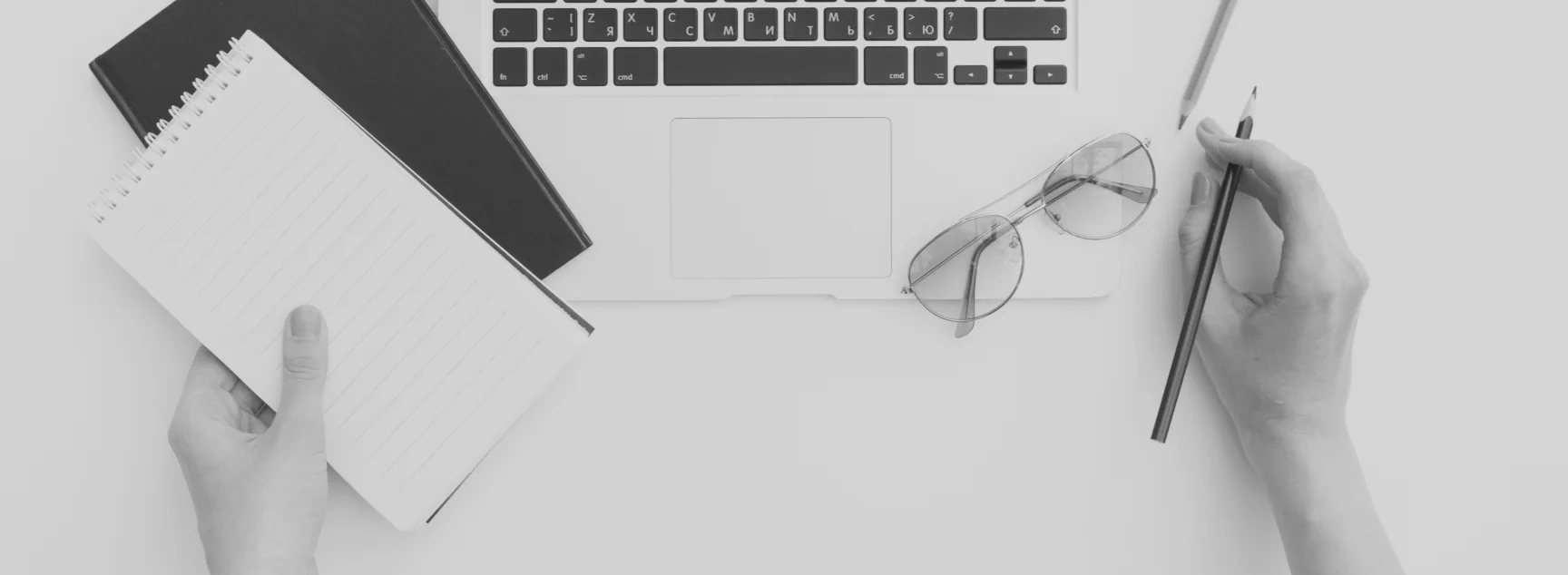
No Bad Questions About ML
Definition of Augmented analytics
What is augmented analytics?
Augmented analytics is a form of business intelligence (BI) that leverages artificial intelligence (AI) and machine learning (ML) techniques to automate tasks, enhance insights, and make data analysis more accessible to a wider range of users. It augments traditional BI capabilities by providing automated data preparation, data discovery, and predictive analytics.
Business users and executives find augmented analytics benefits, simplifying the process of extracting value from data, even for those without extensive technical skills. These technologies enable users to locate relevant information easily, pose insightful questions, and swiftly uncover meaningful insights tailored to their business context.
In addition to empowering non-experts, augmented analytics software also accelerates and enhances the work of analysts and advanced users, allowing them to perform more detailed analysis and data preparation tasks more efficiently.
How do augmented analytics work?
Augmented analytics leverages advanced technologies to enhance data analysis processes and make insights more accessible. It usually involves these key operations:
- Data preparation
AI-augmented analytics tools automatically clean, integrate, and preprocess data from various sources, reducing manual effort and errors. - Advanced analytics
Machine learning algorithms analyze data patterns, identify trends, and generate predictive insights. - Natural language processing (NLP)
NLP interfaces enable users to interact with data using natural language queries, making asking complex questions and getting understandable answers easier. - Automated insights
The system generates actionable insights and visualizations, often with explanations and recommendations, without requiring deep technical knowledge from users. - Self-learning
The system continuously learns from user interactions and feedback to improve the accuracy and relevance of its recommendations.
What is the difference between augmented analytics and business analytics?
While both augmented analytics and business analytics involve the analysis of data, there are some key differences:
- Business analytics focuses on traditional methods of analyzing historical data to provide insights and support decision-making. It typically involves manual data preparation and statistical analysis.
- Augmented analytics enhances traditional business analytics by incorporating AI and machine learning. It automates data preparation, provides predictive and prescriptive insights, and uses natural language interfaces to make data analysis more accessible and interactive.
How to implement augmented analytics?
Implementing augmented analytics involves several key steps:
- Identify use cases
Determine the specific business problems or questions that you want to address with augmented analytics. - Select tools and platforms
Choose augmented analytics tools or platforms that align with your specific needs and budget. Popular augmented analytics tools include Tableau with Einstein Analytics, Microsoft Power BI with Azure AI, and Google Cloud's BigQuery with ML capabilities. - Prepare your data
Ensure that your data is clean, consistent, and accessible. Connect and integrate various data sources to ensure a comprehensive analysis. This includes data from CRM systems, databases, and external sources. - Train and deploy models
Develop and deploy AI and ML models that can be used to automate data analysis tasks and generate insights. - Integrate with existing systems
Integrate your augmented analytics solution with your existing BI tools and business applications. - Monitor and optimize
Continuously monitor the performance of the analytics tools, gather user feedback, and make necessary adjustments to improve accuracy and relevance. - Provide training and support
Provide training and support to your users to help them effectively leverage augmented analytics capabilities.
Key Takeaways
- Augmented analytics revolutionizes data analysis by integrating AI and machine learning, making it easier for both business users and analysts to derive actionable insights.
- It improves efficiency and accessibility, distinguishing itself from traditional business analytics through automation and advanced analytics capabilities.
- Implementing augmented analytics involves careful selection of tools, data preparation, and continuous optimization to maximize benefits and enhance decision-making processes.