The financial services industry is undergoing a deep transformation powered by artificial intelligence. Unlike earlier waves of digitalization that focused on automating what already existed, AI-first products are about rethinking financial services from the ground up.
According to the World Economic Forum's white paper "Artificial Intelligence in Financial Services," financial institutions spent $35 billion on AI in 2023, with projections reaching $97 billion by 2027. McKinsey's report "Extracting value from AI in banking" confirms that banks recognize AI as more than just an efficiency play—it's a strategic imperative that can redefine competitive advantage and transform entire business domains.
When AI is built into the foundation — rather than added on top of outdated systems — it opens the door to entirely new levels of value, efficiency, and personalization. Forward-thinking companies are using this approach to deliver smarter, faster, and more relevant experiences to their customers.
In this article, we will explore how AI-first financial products are reshaping key areas of financial services, discuss strategic advantages and challenges and outline practical steps for institutions aiming to leverage AI effectively to achieve transformative outcomes.
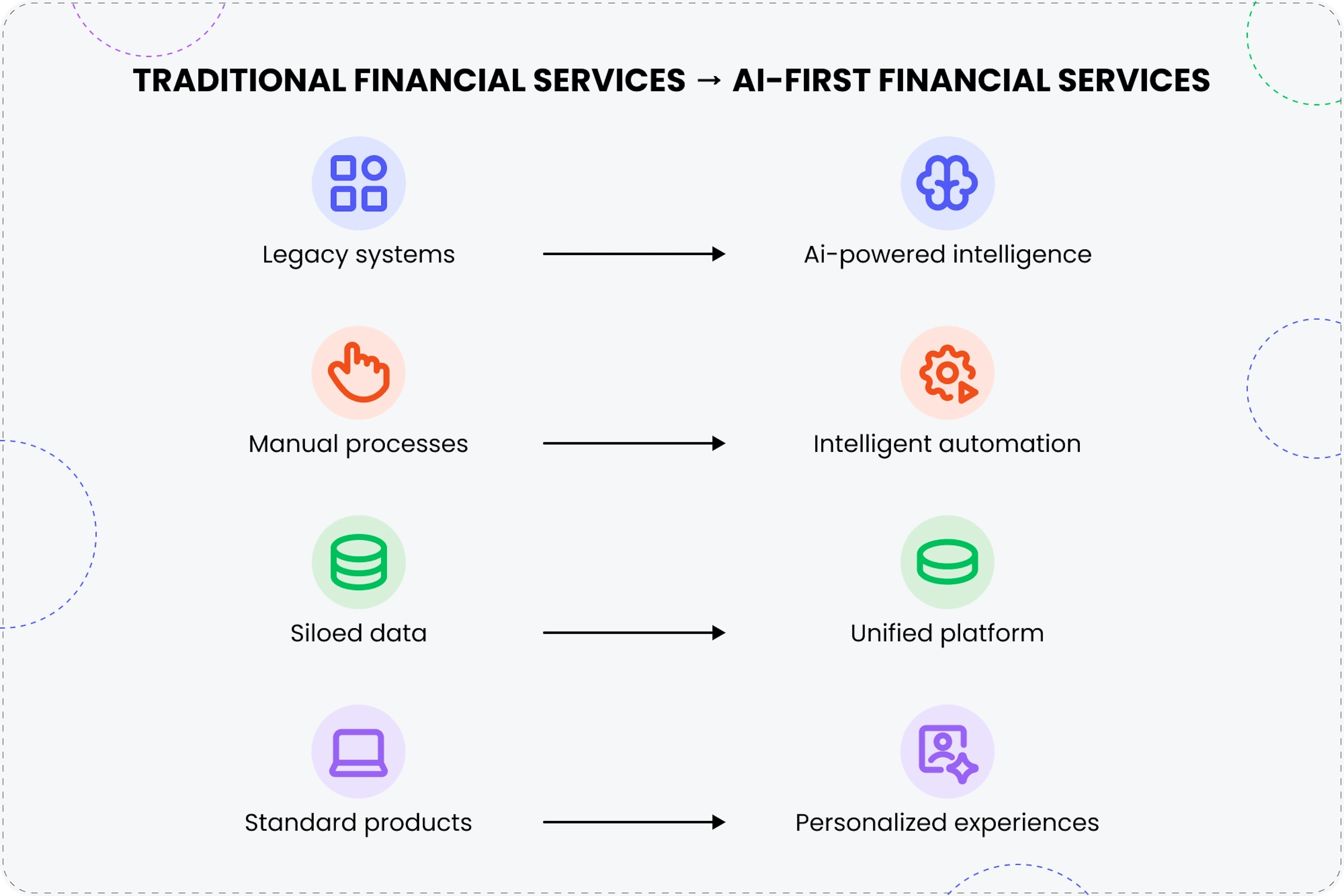
From AI as a feature to AI as the foundation
The latest McKinsey Global Survey on AI shows that more organizations across industries are adopting this technology. However, most organizations are still testing AI or using it only in limited ways, not broadly across all business areas. For an in-depth look at digital transformation strategies in finance, explore our detailed insights here:
Yet, a few leading banks have successfully implemented AI across their entire organizations, including advanced generative AI, and are starting to see significant benefits.
There are two fundamentally different approaches to incorporating AI in financial services:
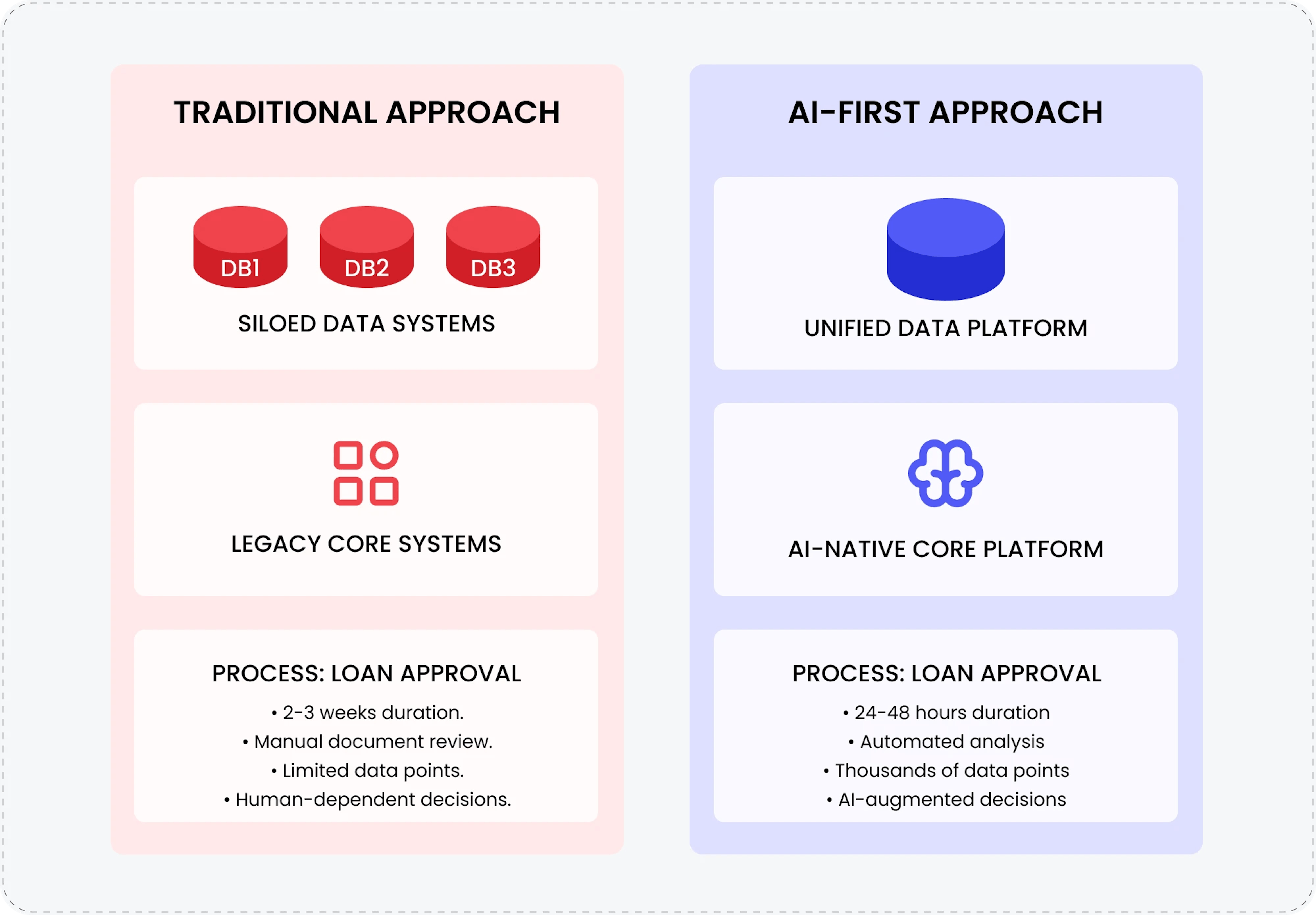
Traditional approach
Established financial institutions typically add AI to their existing systems as an enhancement or additional feature. The McKinsey report highlights how this approach severely limits innovation potential because these organizations face significant constraints:
Siloed data architectures
In traditional banks, customer data is stored in separate, disconnected systems. For example, your checking account data might be in one system, credit card information in another, mortgage details in a third, and investment information in yet another. These systems often don't communicate well with each other.
When a bank wants to implement AI for personalized financial advice, it struggles because the AI can't easily access a complete picture of your financial situation. It's like trying to solve a puzzle with pieces scattered across different rooms.
Legacy technology stacks
Many financial institutions run on core banking systems that were built decades ago, often using COBOL or other legacy programming languages. These systems were designed well before modern AI was conceived.
For instance, a bank might run its deposit accounts on a mainframe system from the 1980s. This system wasn't designed with APIs or real-time data processing capabilities that modern AI requires. Connecting a sophisticated AI system to these old technologies is extremely complex and often requires extensive custom middleware, limiting what the AI can actually do.
Organizational silos
In traditional financial institutions, business units (like retail banking, wealth management, and commercial lending) operate independently from the technology department.
When implementing AI, this creates problems because the business teams define what they want without understanding technological possibilities, while the technology teams build solutions without a deep understanding of customer needs or business processes. For example, the wealth management division might request an AI chat assistant without collaborating with the technology team on how it could be integrated with customer portfolio data.
Risk-averse cultures
Financial institutions are naturally conservative due to regulatory requirements and the importance of maintaining trust. This typically leads to a preference for small, incremental improvements rather than bold reinvention.
For instance, rather than reimagining the entire loan approval process with AI at its core, a bank might just add an AI document scanner to the existing manual workflow. While this improves efficiency slightly, it doesn't transform the experience or dramatically reduce approval times as a fully AI-driven process could.
These constraints make it difficult for traditional financial institutions to fully leverage AI's potential. By contrast, companies building AI-first financial products without these legacy constraints can design fundamentally new experiences and capabilities from the ground up.
AI-first approach
By contrast, designing financial products with AI as their foundation from the beginning opens entirely new possibilities. This approach starts with questions like:
- What if we designed a lending product around AI's ability to process thousands of data points rather than just credit scores?
- What if investment management began with AI's pattern recognition abilities rather than traditional portfolio theory?
- What if customer service was built around conversational AI rather than trying to add chatbots to existing call centers?
The difference is similar to the distinction between adding electric motors to horse carriages versus designing automobiles from scratch. The former improves an existing system incrementally; the latter creates something transformative that wasn't possible before.
AI-first financial products exhibit several distinctive characteristics:
- Core AI functionality — AI isn't a feature but the fundamental technology powering the product's value proposition
- Continuous learning and adaptation — Products improve automatically through usage
- Orchestrated intelligence — Services that coordinate multiple specialized AI agents to complete complex tasks
- Anticipatory design — Solutions that predict user needs rather than merely responding to requests
- Natural interfaces — Conversational, intuitive interactions that minimize friction
AI-first design redefines what's possible in financial services. But vision alone isn't enough — organizations must also re-engineer the processes behind the products.
This is where AI delivers tangible value: by transforming the way core financial operations are run.
Transforming processes with AI
AI dramatically reshapes key financial processes by automating repetitive tasks and enhancing decision-making capabilities:
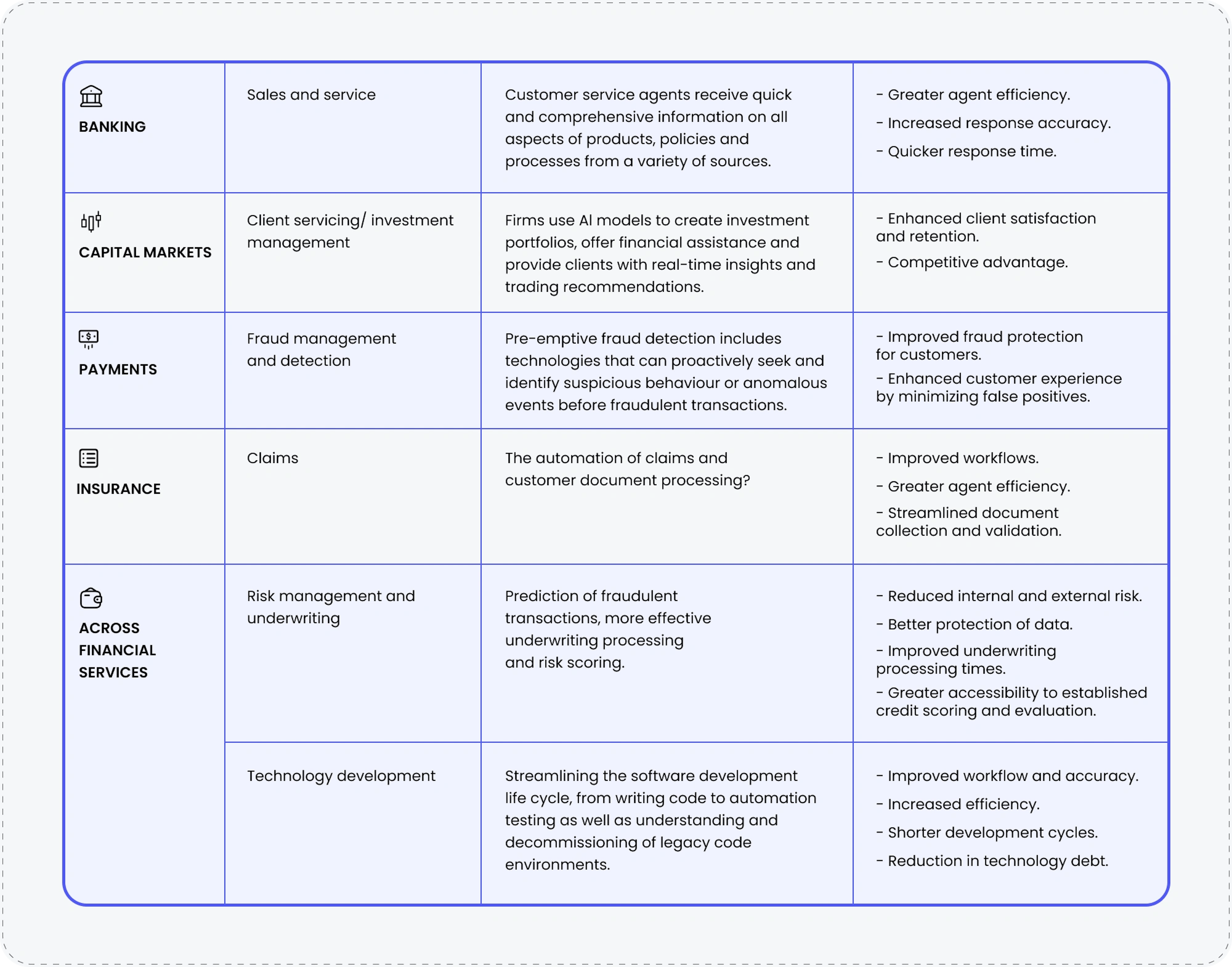
The adoption of AI-first approaches varies across different sectors of financial services, each finding unique applications that address their specific challenges:
1. Autonomous credit assessment systems
Traditional credit scoring relies on limited historical data points and static models. AI-first lending platforms fundamentally transform this by:
- Evaluating thousands of alternative data signals to assess creditworthiness
- Adapting underwriting criteria based on real-time economic conditions
- Predicting future financial health rather than simply examining past behavior
- Customizing financing terms based on granular risk assessment
McKinsey's report highlights how this transformation can occur:
With orchestrated multiagent systems, agents can handle most credit underwriting tasks. A credit manager steps in to review the agents' output and handle tasks that require the human touch: chatting with the customer, visiting the small business in question, and presenting the credit offer to the customer.
The result is extraordinary efficiency and effectiveness. According to McKinsey, analyzing the effects of using multiagent systems to prepare credit memos showed "credit analyst productivity gains of 20 to 60 percent, depending on various factors, and roughly 30 percent faster decision making."
2. Hyperintelligent financial planning
Traditional financial planning has been reactive and template-driven. AI-first planning tools transform this experience by:
- Continuously monitoring account activity, market conditions, and economic indicators
- Predicting future cash flow challenges before they materialize
- Suggesting proactive adjustments to spending, saving, and investing patterns
- Simulating the potential impact of major life decisions using personalized financial data
The World Economic Forum report notes that leading banks are "using AI to help with decision-making, significantly enhancing productivity by building the architecture required to generate real-time analytical insights and translating them into messages addressing precise customer needs."
3. AI-orchestrated wealth management
Traditional wealth management is constrained by human advisor capacity and often lacks personalization at scale. AI-first wealth platforms:
- Deploy multiple specialized AI agents to handle different aspects of wealth management
- Provide institutional-grade investment capabilities to mass-affluent customers
- Create dynamic asset allocations that adapt to changing market conditions
- Offer conversational interfaces that explain complex financial concepts in personalized ways
McKinsey notes that within private banking, relationship management for high-net-worth clients is one of the key subdomains being transformed by AI, where AI agents can handle everything from client risk profiling to portfolio management.
4. Dynamic risk management ecosystems
Traditional risk management relies on periodic manual assessments and static thresholds. AI-first risk systems:
- Continuously monitor thousands of risk indicators in real-time
- Autonomously adjust risk parameters based on emerging patterns
- Simulate complex market scenarios using real-time data
- Predict potential fraud attempts before they occur
The World Economic Forum report highlights risk management as one of the most promising areas for AI application, where AI can help with "prediction of fraudulent transactions, more effective underwriting processing and risk scoring."
Key advantages and challenges of AI-first product development
Organizations that build financial products with AI as the foundation rather than an add-on enjoy several competitive advantages:
➕ Significant reduced technical debt
AI-first development allows teams to avoid the constraints of legacy systems and outdated infrastructure. This significantly reduces technical debt and creates room for more scalable, future-ready solutions. McKinsey notes that traditional banks often struggle with "the presence of legacy technology infrastructure that may not be compatible with more modern AI solutions." With an AI-first mindset, companies can move faster and stay flexible.
➕ Faster iteration and improvement
These products can evolve rapidly based on real-world usage and feedback. The data collected feeds back into the AI models, creating a virtuous cycle of continuous improvement that legacy systems rarely achieve.
➕ Seamless end-to-end experiences
Rather than patching together various technologies, AI-first products can provide seamless experiences where every component works together harmoniously. This creates dramatically improved customer experiences compared to traditional offerings.
➕ Novel problem-solving approaches
AI-first products can solve problems that would be impossible with traditional approaches. For example, multiagent systems can manage complex financial workflows that would otherwise require multiple human specialists, making sophisticated financial services accessible to broader customer segments.
Despite the tremendous potential, building AI-first financial products comes with challenges:
➖ Complex regulatory landscape
AI introduces new questions around transparency, accountability, and fairness. The regulatory landscape isn't just complex—it's actively evolving in response to AI implementation. Financial institutions face a multi-layered challenge:
- Financial regulations vary widely across countries and are constantly changing
- AI systems must be explainable for audit purposes, limiting the use of certain advanced models
- Compliance requirements create significant overhead and slow down innovation
➖ Data access, privacy, and ethics
Financial data is both sensitive and limited. Companies can't easily share information about fraudulent transactions or loan defaults, yet this data is essential for building effective AI.
Also, financial markets and consumer habits change constantly, making AI models become outdated much faster than in other industries. At the same time, strict privacy laws limit what data companies can use, creating a difficult balance between building effective AI systems and following regulations.
➖ Shortage of specialized talent
Developing AI-first products requires rare expertise at the intersection of machine learning, deep understanding of financial markets, risk management, and regulatory compliance and product development. Attracting and retaining this talent is highly competitive, and internal upskilling takes time.
➖ Maintaining security and customer trust
Financial AI systems operate in an environment where mistakes carry significant consequences. Customers are cautious about letting AI handle their money. AI systems must operate in high-stakes environments where even small errors can have outsized impacts. In other ways, it can accidentally create unfair outcomes if it learns from biased historical data, creating both ethical and legal problems.
Building AI-first financial products: strategic framework
For organizations looking to develop AI-first financial products, both reports suggest a strategic framework:
Choose the right scope of transformation
McKinsey advises against "letting a thousand flowers bloom with many disparate, siloed AI projects." Instead, financial institutions should:- Identify specific business domains and subdomains to transform end-to-end
- Focus on areas with high business impact and technical feasibility
- Develop reusable components that can be applied across multiple domains
- Target subdomains that can drive 70-80% of total incremental value from AI
The key distinction with AI-first approaches is their comprehensive nature. Rather than applying AI to optimize existing processes, these initiatives reimagine entire business domains from first principles, considering what would be possible if designed specifically to leverage AI capabilities.Implement a full-stack approach
Building successful AI-first products requires investment across the entire AI capability stack:- Combine generative AI with traditional analytics and digital tools
- Build robust data foundations with both structured and unstructured data
- Implement machine learning operations capabilities for scale
- Create reusable AI components and patterns
- Establish appropriate governance frameworks
Unlike traditional technology implementations that might focus primarily on front-end applications, AI-first approaches require integration across all layers of the technology stack. This comprehensive approach enables capabilities impossible with more limited implementations.Organize your team for AI-powered innovation
The organizational approach must evolve to support AI-first product development:- Create cross-functional teams blending business, tech, and AI expertise
- Establish an AI control tower to coordinate enterprise decisions
- Adopt agile ways of working that enable rapid iteration
- Adopt agile ways of working that enable rapid iteration
- Implement value capture mechanisms to track transformation outcomes
- Build a culture of continuous learning and adaptation
AI-first product development requires breaking down traditional organizational boundaries between business and technology functions. Rather than business teams defining requirements for technology teams to implement, these cross-functional teams collaboratively explore possibilities enabled by AI capabilities while ensuring alignment with business objectives.Balance innovation with responsible AI
Establish governance frameworks for AI use cases and data models:- Implement robust risk protocols and controls
- Ensure compliance with evolving regulations
- Address issues of transparency, bias, and ethics
- Build customer trust through responsible practices
Responsible AI isn't an afterthought in AI-first product development but a fundamental design principle. These products incorporate explainability, fairness, and transparency at their foundation rather than attempting to add these features retrospectively.
The World Economic Forum report notes that "84% of financial organizations are implementing or planning a framework to govern how AI will be built, trained, used and audited, to adhere to business principles and relevant regulations."
Moving towards an AI-powered future
To realize these transformative changes, financial leaders must adopt a dynamic AI strategy that incorporates continuous technology monitoring and adaptation. Over the next two to three years, key technology advancements will be particularly influential:
Small language models (SLMs)
An SLM is a machine-learning algorithm that has been trained on a dataset that is smaller and more specific than those of large language models (LLMs). SLMs are typically deployed for a single specific task (for example, answering customers' questions about a particular product); they can be more efficient and faster than LLMs due to their size and their use of a more targeted dataset.
These specialized models enable AI-first financial products to deliver expertise in specific domains with greater efficiency and reliability than general-purpose systems. For example, an AI-first mortgage product might employ specialized models for property valuation, borrower qualification, and regulatory compliance rather than relying on a single general-purpose system.
Retrieval-augmented generation (RAG)
RAG is a process that enhances the capability of generative AI models by optimizing LLMs—increasing both accuracy and reliability. This process uses in-house data repositories (internal organizational data such as policy or procedural documents) to support the validation of responses to ensure it is combining the latest and most accurate responses. Companies can incorporate RAG into their AI-based assistants, making them more effective and reliable at answering customer questions, by validating responses against a master repository (such as knowledge articles in a repository).
This capability is crucial for AI-first financial products operating in regulated environments where accuracy is paramount. By integrating retrieval mechanisms directly into their architecture, these products can provide responses that combine the fluency of generative AI with the reliability of verified information.
AI agents
These are designed and built to understand inputs, make decisions, and act on them without any human intervention. When AI acts as an agent instead of a service, it is no longer locked into assisting with a single task or function but can be applied to a broader range of problems and decision-making opportunities. An example of this is the use of AI agents that can process enquiries, action customer requests and make product recommendations. These financial chatbots and virtual assistants must constantly adapt to changing customer behavior patterns and market conditions.
AI-first financial products increasingly incorporate agent capabilities to handle end-to-end processes rather than just individual tasks. For example, an AI-first financial planning solution might dynamically adjust investment recommendations, initiate transactions, and coordinate with tax planning tools—all autonomously within established parameters.
Quantum computing
Quantum computers can solve certain complex problems much faster than regular computers… While conventional computers use bits (0s and 1s), quantum computers use quantum bits or "qubits" that can exist in multiple states simultaneously.
When combined with AI in financial services, they can process huge amounts of financial data in seconds, spot hidden patterns in transaction data, and make complex decisions almost instantly. For example, a bank could quickly identify fraudulent transactions among millions of legitimate ones by analyzing patterns that would take regular computers hours or days to find.
While this technology is still developing, it will eventually allow financial products to perform calculations that are simply impossible with today's computers, transforming risk analysis, investment strategies, and fraud prevention. Companies like JPMorgan Chase and Goldman Sachs have started quantum computing research programs to prepare for this future capability.
To wrap up
AI-first financial products represent a fundamental reimagining of financial services. The organizations that will lead this transformation are those that recognize AI not merely as a technology implementation but as a strategic foundation that changes how they conceive of financial products and services. The future belongs not to those who simply adopt AI, but to those who reimagine their entire approach to product development with AI at its core to create solutions that are more personalized, efficient, and powerful than previously possible.
Those who successfully implement the strategic framework outlined here—choosing the right transformation scope, taking a full-stack approach, organizing for AI-powered innovation, and balancing innovation with responsibility—will find themselves positioned to create disruptive solutions that redefine customer expectations and competitive dynamics.
Ready to transform your financial products with AI-first solutions? Mad Devs specializes in helping financial institutions reimagine their offerings with cutting-edge AI at the core. Contact us today to discuss how we can help you build the next generation of financial services that deliver unprecedented value to your customers.