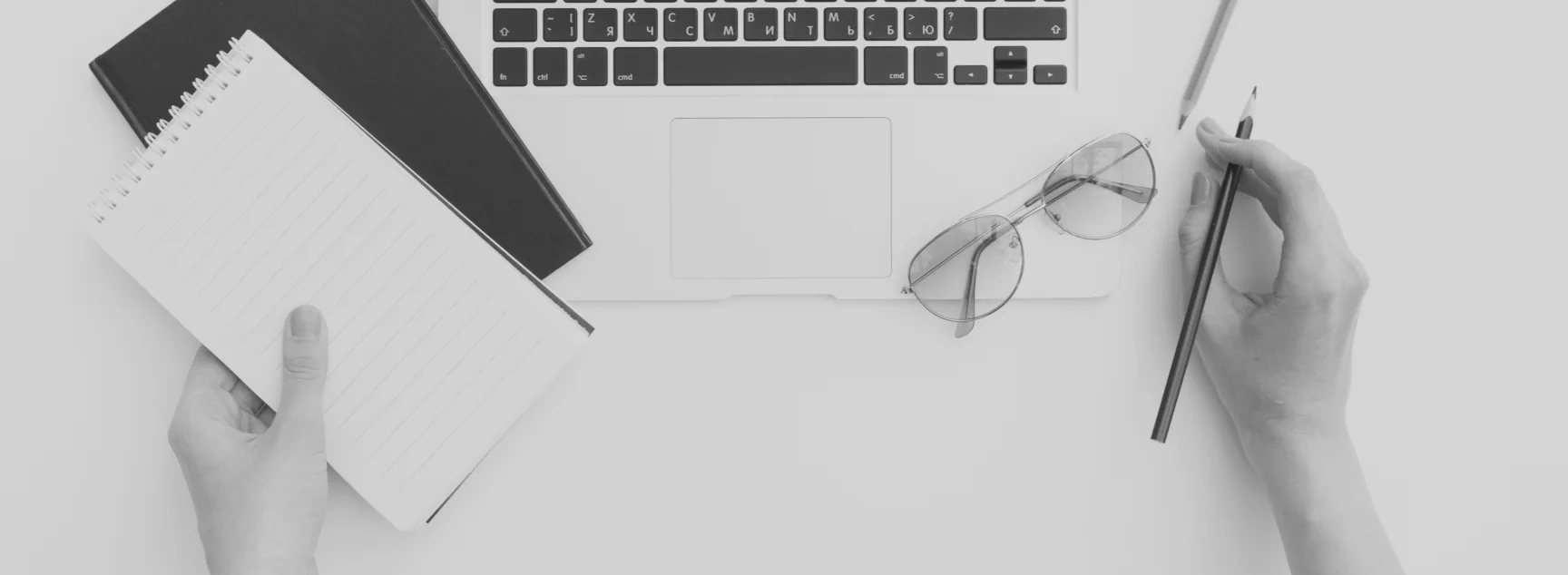
No Bad Questions About ML
Definition of Edge computing
How does edge computing work
Edge computing brings processing power and data storage closer to the source of the data, unlike traditional cloud computing, which moves everything to a central data center.
Edge deployments come in various forms but generally fall into two main categories:
1. Upstream applications. Focus on collecting data from sensors and devices, then filtering and transmitting relevant information to central data centers.
2. Downstream applications. Prioritize delivering data to end users with minimal latency (delay), ideal for real-time experiences like video streaming or online gaming.
Edge vs. cloud vs. fog computing
Here's a quick breakdown:
- Cloud computing ā Centralized data processing in large data centers, offering vast storage and processing power but with higher latency for geographically distant devices.
- Edge computing āĀ Processing happens at the network's "edge," closer to data sources, ideal for real-time needs and bandwidth limitations.
- Fog computing ā Sits between edge and cloud, often acting as a local processing layer that aggregates data from edge devices before sending it to the cloud. It can also provide additional processing power for edge devices.
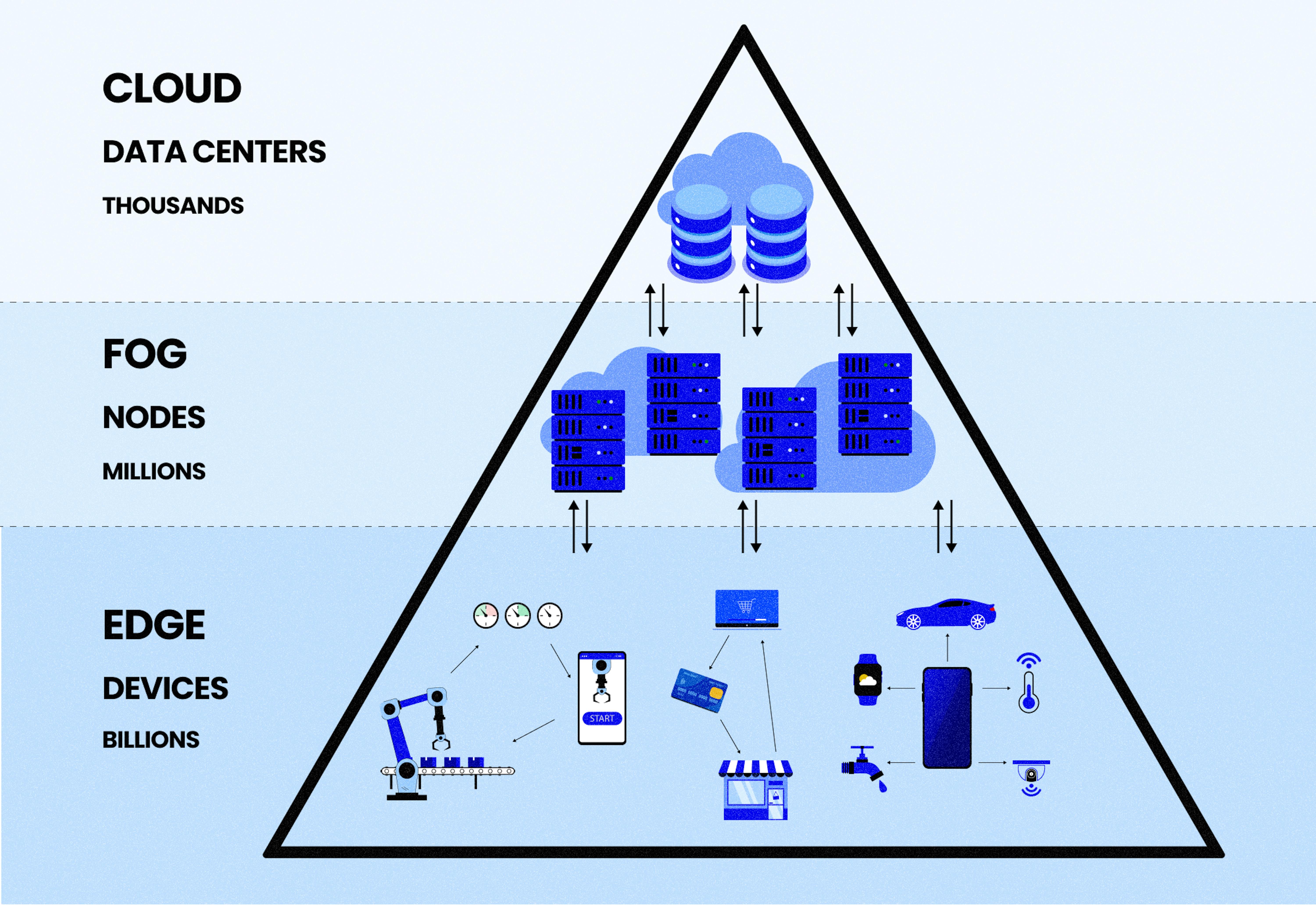
Why is edge computing important
Edge computing is gaining popularity as it enables companies to gather and analyze data faster. With quick access to data, organizations can make better decisions about their operations and business functions. When used effectively, edge computing can enhance safety, automate processes, and enhance user experience.
What are edge computing benefits?
The main benefits are:Ā
- Data processing locally instead of sending it back and forth to the cloud reduces latency (delay), which is crucial for real-time applications like factory automation or self-driving cars.
- Less data needs to travel long distances, saving on bandwidth costs and improving network efficiency.
- Some edge devices can still function even when disconnected from the cloud, enabling local processing and decision-making.
Edge computing use cases
The power of edge computing, with its lightning-fast speeds and simple setup, is revolutionizing how businesses operate across various sectors. Here are some prime examples:
Manufacturing ā boosts efficiency with real-time data for automation, on-site analysis, and optimized machine communication.
Self-driving cars āĀ Enables instant decisions with local processing and car-to-car communication for safety and responsiveness.
Energy sector ā Improves safety and efficiency with real-time data analysis for hazard detection, resource allocation, and maintenance prediction.
Healthcare ā Enhances privacy and reduces data transfer by storing critical patient data locally and processing it closer to the source.
Key Takeaways
- Edge computing brings data processing and storage closer to where data is generated, unlike traditional cloud computing, which relies on centralized data centers.
- It can be categorized into two main types: upstream applications, focusing on data collection and transmission to central data centers, and downstream applications, prioritizing real-time data delivery to end-users.
- Compared to cloud and fog computing, edge computing offers faster data processing suitable for real-time needs and bandwidth limitations.
- It's essential because it enables quick data analysis, leading to better decision-making, improved safety, process automation, and enhanced user experiences.
- Key benefits of edge computing include reduced latency, minimized data travel distances, cost savings on bandwidth, and the ability for some devices to function independently of cloud connectivity.
- Edge computing finds applications across various industries, such as manufacturing, self-driving cars, energy, and healthcare. It revolutionizes operations by providing real-time data insights, automation, and enhanced safety measures.