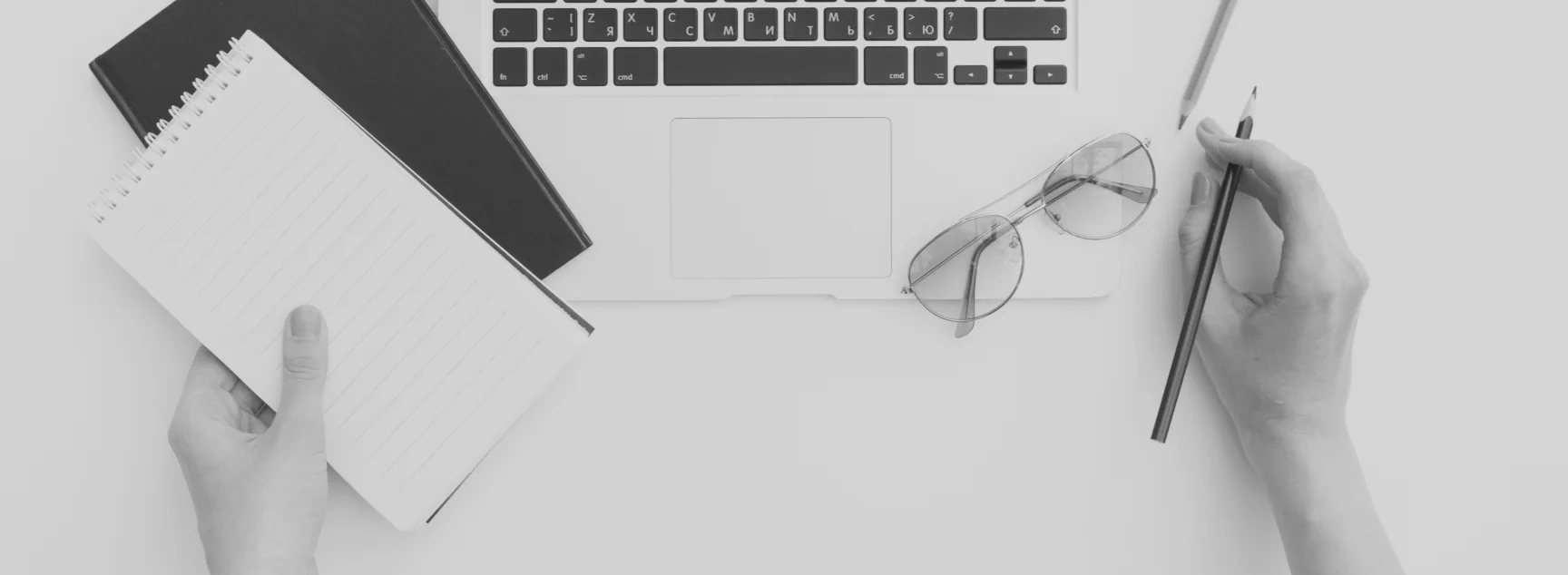
No Bad Questions About ML
Definition of Cognitive automation
What is cognitive automation?
Cognitive automation is the use of artificial intelligence (AI), machine learning (ML), and natural language processing (NLP) to automate complex business processes that require reasoning, decision-making, and learning from data. It differs from traditional robotic process automation (RPA) with predefined rules in that cognitive automation can analyze unstructured data and adapt to changing conditions.
Cognitive automation enables systems to process vast amounts of information, identify patterns, and improve performance over time. This makes it particularly useful for tasks that involve judgment, such as fraud detection, customer service, and document processing. It reduces the need for human intervention in repetitive and knowledge-based tasks to allow employees resources to focus on higher-value activities. As businesses increasingly adopt AI-driven solutions, cognitive automation is becoming a critical component of digital transformation strategies.
How does cognitive automation work?
Cognitive automation works by creating AI-driven technologies such as natural language processing, machine learning, and computer vision that simulate human cognitive functions. These tools process and analyze both structured and unstructured data to extract insights from sources like emails, documents, and voice recordings. The system applies predefined rules combined with AI models to:
- Make decisions
- Detect anomalies
- Predict outcomes
Over time, machine learning algorithms help improve accuracy by learning from past interactions and feedback. Cognitive automation can also integrate with enterprise software, such as customer relationship management (CRM) or enterprise resource planning (ERP) systems, to enhance process automation. Through a combination of automation and intelligence, it enables businesses to optimize operations and provide more responsive services.
What is the advantage of cognitive automation?
The main advantage of cognitive automation is its ability to handle complex, non-routine tasks that require reasoning and adaptability without the necessity of human intervention. This alone reduces significant manual effort and operational costs. Other advantages include:
- Minimizing human errors and improving accuracy
- Accelerating decision making
- Enabling more intelligent and context-aware interactions with customers
As a result, businesses that implement cognitive automation gain a competitive edge with an increase in efficiency, improvements in service quality, and the freeing up of human workers for strategic tasks.
What is an example of cognitive automation?
An example of cognitive automation is an AI-powered customer service chatbot that understands and responds to natural language queries, processes support tickets, and learns from interactions to improve its responses over time. Unlike simple rule-based bots, cognitive automation chatbots analyze customer sentiment, detect intent, and provide more personalized and contextually relevant assistance.
Another example is an intelligent document processing system that automatically extracts key information from invoices, contracts, or medical records, reducing the need for manual data entry. In financial services, cognitive automation can be used for fraud detection by analyzing transaction patterns and identifying suspicious activities in real time. In healthcare, AI-powered diagnostic tools assist doctors by analyzing medical images and patient records to provide accurate recommendations. These examples illustrate how cognitive automation can enhance efficiency, accuracy, and decision-making across various industries.
Key Takeaways
- Cognitive automation is the use of AI, ML, and NLP to automate complex business processes that require reasoning, decision-making, and learning from data.
- Unlike traditional robotic process automation that acts with predefined rules, cognitive automation can analyze unstructured data and adapt to changing conditions.
- Functionality includes processing unstructured data, recognizing patterns, making decisions, and predicting outcomes.
- Advantages include increased efficiency, reduced errors, faster decision-making, and improved customer experience.
- Examples include AI chatbots for customer service and intelligent document processing systems.